Synthetic Data for Artificial Intelligence and Machine Learning: Tools, Techniques, and Applications II
Chair welcome and introduction
22 April 2024 • 5:00 PM - 5:05 PM EDT
DoD's microelectronics for the defense and commercial sensing ecosystem (Plenary Presentation)
Presenter(s): Dev Shenoy, Principal Director for Microelectronics, Office of the Under Secretary of Defense for Research and Engineering (United States)
22 April 2024 • 5:05 PM - 5:45 PM EDT
NATO DIANA: a case study for reimagining defence innovation (Plenary Presentation)
Presenter(s): Deeph Chana, Managing Director, NATO Defence Innovation Accelerator for the North Atlantic (DIANA) (United Kingdom)
22 April 2024 • 5:50 PM - 6:30 PM EDT
The CHIPS Act Microelectronics Commons network is accelerating the pace of microelectronics technology development in the U.S. This panel discussion will explore opportunities for crossover from commercial technology into DoD systems and applications, discussing what emerging commercial microelectronics technologies could be most impactful on photonics and sensors and how the DoD might best leverage commercial innovations in microelectronics.
Moderator:
John Pellegrino, Electro-Optical Systems Lab., Georgia Tech Research Institute (retired) (United States)
Panelists:
Shamik Das, The MITRE Corporation (United States)
Erin Gawron-Hyla, OUSD (R&E) (United States)
Carl McCants, Defense Advanced Research Projects Agency (United States)
Kyle Squires, Ira A. Fulton Schools of Engineering, Arizona State Univ. (United States)
Anil Rao, Intel Corporation (United States)
Moderators:
Kimberly E. Manser, DEVCOM C5ISR (United States)
Panelists:
Raghuveer Rao, Army Research Laboratory
Colin Reinhardt, Naval Intelligence Warfare Center
Corban Rivera, Johns Hopkins University, Applied Physics Laboratory
Sek Chai, Latent AI
Alex Hauptmann, Carnegie Mellon University
Poster Setup: Tuesday 12:00 PM - 5:30 PM
Poster authors, view poster presentation guidelines and set-up instructions at http://spie.org/DCSPosterGuidelines.
Welcome and opening remarks
24 April 2024 • 8:30 AM - 8:40 AM EDT
Army intelligence data and AI in modern warfare (Plenary Presentation)
Presenter(s): David Pierce, U.S. Army Intelligence (United States)
24 April 2024 • 8:40 AM - 9:20 AM EDT
FUTUR-IC: A three-dimensional optimization path towards building a sustainable microchip industry (Plenary Presentation)
Presenter(s): Anu Agarwal, Massachusetts Institute of Technology, Microphotonics Ctr. and Materials Research Lab. (United States)
24 April 2024 • 9:20 AM - 10:00 AM EDT
View call for papers
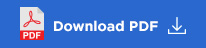
What you will need to submit:
- Presentation title
- Author(s) information
- Speaker biography (1000-character max including spaces)
- Abstract for technical review (200-300 words; text only)
- Summary of abstract for display in the program (50-150 words; text only)
- Keywords used in search for your paper (optional)
- Check the individual conference call for papers for additional requirements (i.e. extended abstract PDF upload for review or instructions for award competitions)